A Working Example of NLP That You Can Use Today
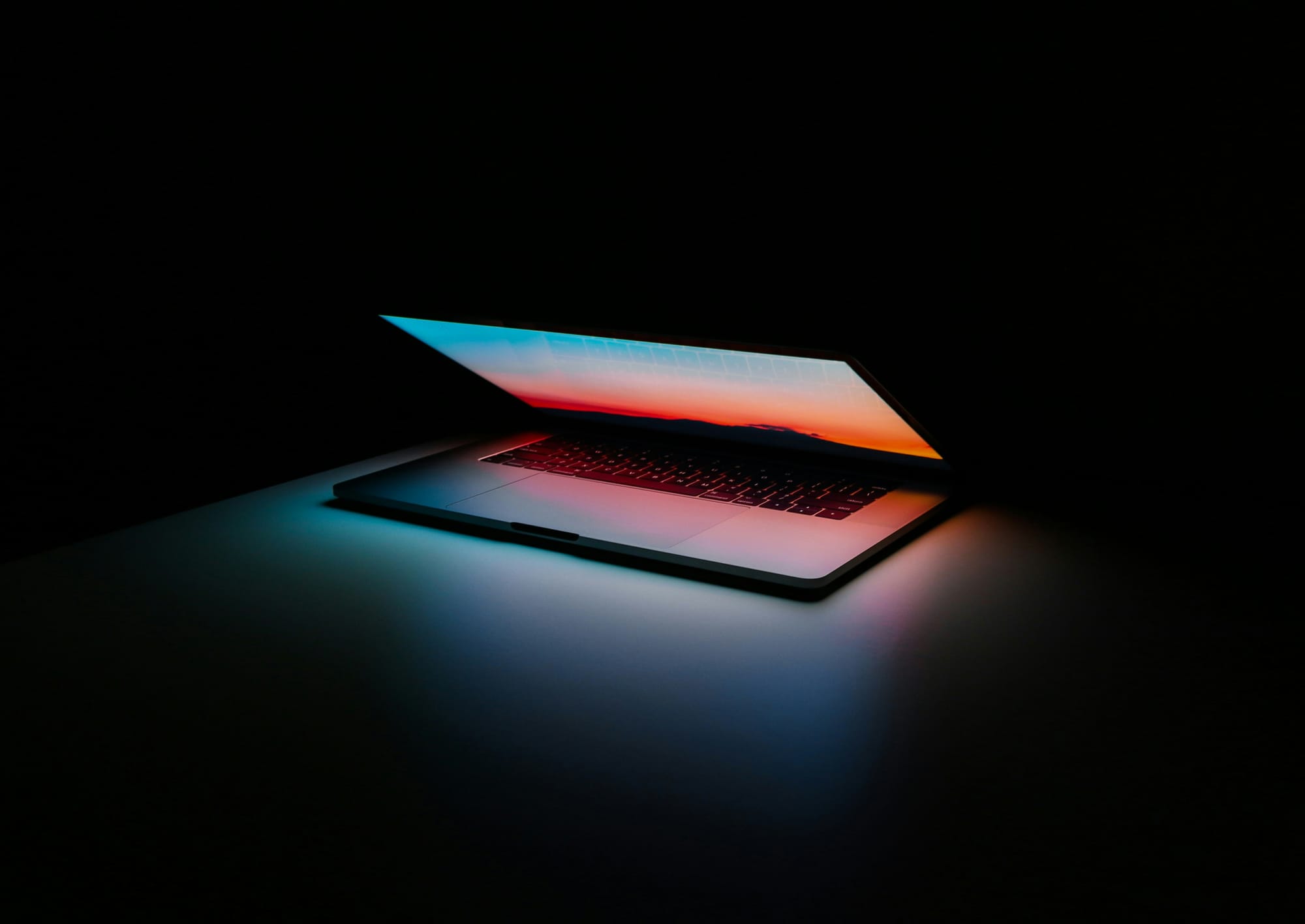
Natural Language Processing (NLP) has made its way into so many areas of our lives. From search engines that seem to understand what we’re looking for to autocorrect that turns typos into sensible text (well, mostly), NLP is an unsung hero in modern tech. But despite its popularity, NLP can still feel like an overwhelming field, especially if you’re just starting out.
I’ve been in tech long enough to appreciate how powerful NLP can be, but also how frustrating it is when it doesn’t quite get things right. A chatbot that misunderstands a question or an autocorrect that takes you in the wrong direction – we’ve all been there. That said, with a bit of understanding and some well-chosen tools, NLP can be accessible and genuinely useful. So, let’s dive into a working example that you can implement today.
Real-World Use Case: Sentiment Analysis for Customer Feedback
Let’s imagine you work at a small company that receives feedback from customers. You have a growing number of reviews, support tickets, and emails, but you’re a small team and can’t manually read each message. You’d love to know if most customers are happy, neutral, or dissatisfied so you can prioritise responses.
Sentiment analysis, one of the most popular NLP applications, is an ideal solution here. With sentiment analysis, we can automatically classify feedback as positive, neutral, or negative. This way, you can quickly spot trends and address any recurring issues.
Step 1: Setting Up Sentiment Analysis
To get started, we’ll use Python and the TextBlob
library. TextBlob
is a friendly NLP library that allows you to perform tasks like sentiment analysis without needing advanced NLP expertise.
Install TextBlob
If you haven’t already installed TextBlob
, you can do so using the following command:
pip install textblob
Analysing Sentiment with TextBlob
Let’s start by analysing some sample feedback. Suppose you have a few customer comments stored in a list, and you want to classify each one as positive, neutral, or negative.
Here’s some simple code to get us started:
from textblob import TextBlob
# Sample feedback
feedback_list = [
"I absolutely love this product!",
"It's okay, but I've seen better.",
"Not happy with the service. It took too long to arrive."
]
# Analyse each comment
for feedback in feedback_list:
analysis = TextBlob(feedback)
sentiment_score = analysis.sentiment.polarity
if sentiment_score > 0:
sentiment = "Positive"
elif sentiment_score < 0:
sentiment = "Negative"
else:
sentiment = "Neutral"
print(f"Feedback: '{feedback}'\nSentiment: {sentiment}\n")
Here’s what’s happening in this code:
- We create a
TextBlob
object for each feedback comment. - Using
TextBlob
, we get a sentiment polarity score for each comment. The polarity ranges from -1 to 1, with negative values indicating negative sentiment, positive values indicating positive sentiment, and zero indicating neutral sentiment. - We classify each comment as positive, neutral, or negative based on the score.
Expected Output
Running this code should give you something like:
Feedback: 'I absolutely love this product!'
Sentiment: Positive
Feedback: 'It's okay, but I've seen better.'
Sentiment: Neutral
Feedback: 'Not happy with the service. It took too long to arrive.'
Sentiment: Negative
It’s simple but effective. With this code, you can get a quick sense of customer sentiment without reading every comment manually.
Step 2: Automating Sentiment Analysis for Real-Time Use
Now, let’s take it a step further. Imagine your company’s customer support team uses a system like Zendesk to manage support tickets. By connecting this code to your support system, you could automatically tag tickets based on sentiment, helping your team prioritise negative feedback.
Many platforms offer APIs that allow you to pull data in real time. Here’s how you might set up sentiment analysis for incoming tickets:
import requests
from textblob import TextBlob
# Replace with your actual API endpoint and key
API_ENDPOINT = "https://your-ticketing-system.com/api/tickets"
API_KEY = "your_api_key"
# Get the latest tickets
headers = {"Authorization": f"Bearer {API_KEY}"}
response = requests.get(API_ENDPOINT, headers=headers)
tickets = response.json()
# Analyse each ticket's sentiment
for ticket in tickets:
comment = ticket['comment']
analysis = TextBlob(comment)
sentiment_score = analysis.sentiment.polarity
if sentiment_score > 0:
sentiment = "Positive"
elif sentiment_score < 0:
sentiment = "Negative"
else:
sentiment = "Neutral"
print(f"Ticket ID: {ticket['id']}\nComment: {comment}\nSentiment: {sentiment}\n")
This example assumes your ticketing system has an API that lets you retrieve tickets. While every ticketing system is different, the general idea remains the same: pulling the data, running it through sentiment analysis, and then outputting the results. With a bit of setup, you could even store these results in your system, tagging each ticket by sentiment for future reference.
Step 3: Adding Visualisation
For larger volumes of feedback, visualisation can be helpful. Using libraries like matplotlib
or seaborn
, you can create graphs showing sentiment trends over time. This is particularly useful for spotting shifts in customer satisfaction after a product update or campaign.
Here’s a quick example of how you could visualise the distribution of sentiment scores:
import matplotlib.pyplot as plt
# Sample feedback list with polarity scores
feedback_polarity = [TextBlob(feedback).sentiment.polarity for feedback in feedback_list]
# Classify sentiments
sentiments = ["Positive" if score > 0 else "Negative" if score < 0 else "Neutral" for score in feedback_polarity]
# Plot the sentiment distribution
plt.hist(sentiments, bins=3, color='skyblue', edgecolor='black')
plt.title("Sentiment Distribution of Customer Feedback")
plt.xlabel("Sentiment")
plt.ylabel("Frequency")
plt.show()
This code creates a histogram showing how often each sentiment category appears in your feedback. With a quick glance, you’ll be able to see if positive feedback outweighs negative, or if there’s a spike in negative comments that needs attention.
Real-World Example: Beyond Customer Feedback
Sentiment analysis can be used in so many different contexts beyond customer feedback. For instance, some companies use it to monitor social media for mentions of their brand. In one of my previous roles, we used sentiment analysis to scan Twitter for brand mentions. If a trend of negative sentiment appeared, we’d investigate further to see if there was an emerging issue. This proactive approach allowed us to address concerns before they grew into larger problems.
NLP can even be used in HR to analyse employee feedback or conduct surveys. By anonymising feedback, HR departments can look at trends in morale and take steps to improve workplace culture.
The Limitations: Understanding Nuance
Of course, NLP isn’t perfect, especially when it comes to something as nuanced as human emotion. Language can be tricky – sarcasm, irony, or cultural references can throw off an NLP model. A comment like “Oh, fantastic!” could be genuinely positive or deeply sarcastic, and it’s often the context that makes the difference.
That’s why, even with all its advancements, NLP still benefits from a human touch. Sentiment analysis works best when it’s a starting point rather than the sole decision-maker. It’s about equipping teams with tools that help them spot trends and prioritise responses, not automating empathy.
So, getting started with NLP
Natural Language Processing has come a long way, and tools like TextBlob
make it accessible for everyone – even if you’re not a data scientist. From automating simple tasks to identifying trends in large datasets, NLP can make life easier and improve customer experience. And while it’s not flawless, it’s a step in the right direction.
As you dive into NLP, remember that this technology is here to assist, not replace, human insight. Sentiment analysis, while powerful, should be seen as a support tool that complements the judgement and empathy of the people behind it.
So go ahead, give it a try, and see where NLP can make a difference in your work. Whether you’re looking to enhance customer service or gain insights from data, NLP has practical applications you can implement today – no PhD required.